First, who am I to be writing this article? I worked in growth functions at HubSpot for 5 years on both the growth marketing team and product teams. I also served as head of growth at People.ai building out the product-led growth motion from scratch. Now that we’ve gotten that out of the way…
Imagine this, you find that some aspect of your business has become stagnant, that it has plateaued, so you decide to do some research as to how you can change this and increase your revenue. You begin reading a bunch of blog posts about how you need to run marketing experiments and change up your marketing strategy and you decide, “Sure, why not? It’s supposed to help me grow my business, right?”
You Google “growth experiments” and you get a list of “20 experiments you have to run” and you start running a couple.
A week later, you review the data, and nothing has changed. No huge improvements in your clickthrough rates. No spike in the leads you’ve generated. No new users signing up for your software. No spike in revenue.
“Well… I’ve read most experiments don’t work, so I just have to keep trying.”
So you review the list of experiments you have to do and you run those experiments…but still, none of them work.
What’s happening?
Before you go any further, I talk about a lot of tools and templates in this article. Let me know your email and I’ll send them to you.
People often only share the experimentation wins on social media but when you begin experimenting, you realize that most experiments fail.
This happens because many people run experiments and try hacks they see others doing just for the sake of saying they’re experimenting. In reality, they’re just copying what they see other companies doing. I had to learned this the hard way.
At the end of the day, experimentation and running a/b tests without a growth strategy behind it is a formula for failure. That ad hoc approach to experimentation without a strategy is what I call “growth hacking.” It’s hacky without any strategy in mind.
One of the most important business initiatives at HubSpot, a B2B SaaS company, was transitioning from a marketing-and-sales-heavy business model to a self-service freemium model.
This resulted in the creation of our growth team and a new era of data-driven experimentation at the company. The growth team now owns and manages the freemium funnel from user acquisition to monetization.
HubSpot is a large, fast-growing company with many B2B products and many product teams, so running experiments is a complex process.
So, let’s dive into how we think about experiments, our process in determining the best experiments to run, and how startups can apply the same principles.
I previously gave a talk based on this post and have included the video and slides below.
Why run experiments?
We run experiments to improve the user experience of our product, increase the number of people who sign up for our products, encourage users to engage with the product, and make it simple for users to upgrade to a paid product.
There are many factors that might impact a metric, from overall market sentiment to new products being released to a single blog post published by another unrelated website.
By running controlled experiments, we are able to isolate any improvement or decrease in performance to a single factor and make business and product decisions based on both qualitative and quantitative data.
How do we think about experiments?
We generally run experiments for two reasons, (1) to optimize a conversion rate or (2) to learn how a new variable affects performance.
In both cases, we start by understanding the customer journey.
Then when we run the experiment, we track the experimental cohort holistically.
For example, if we wanted to improve the signup conversion rate, we would look at the cohort’s activation and monetization rate. If we wanted to improve the monetization rate, we would look at the cohort’s retention and churn rate.
The challenge with experiments is many tactics have become overused and saturated which means there isn’t much in terms of first-mover advantage related to growth experiments.
Instead, experiments must be based on a solid hypothesis rooted in a strong understanding of the customer.
From there, we start the discussion for any potential experiments.
“What do we believe to be true that would make this experiment potentially successful?”
HubSpot is a B2B software company so our cohorts aren’t as large as B2C companies such as Facebook, Google, and Airbnb. That means it takes longer for our experiments to reach statistical significance.
Since we don’t have the luxury of collecting large cohorts quickly, we focus on impact, not activity, and apply a fine filter for what we anticipate will be high-impact experiments.
Documentation
Documentation plays a big role in our experimentation process. Writing experiment docs forces us to think through the “why” of an experiment, including what we expect to happen, and determining whether it’s worth doing in the first place.
Our experiment documentation include our research, hypothesis, learning objectives, charts we pulled, why we believe the experiment is worth running, and of course how it impacts key performance indicators (KPIs).
We revisit and revise each experiment document as we conduct research and better understand the question we’re attempting to answer.
We’re 100% transparent about the experiments. Every growth team has an Airtable that’s open for the rest of the company to view.
You can get a copy of the growth experiment template I set up in Airtable here. That template will help with building a roadmap of experiments and project management when you start running multiple experiments at once in different parts of your product.
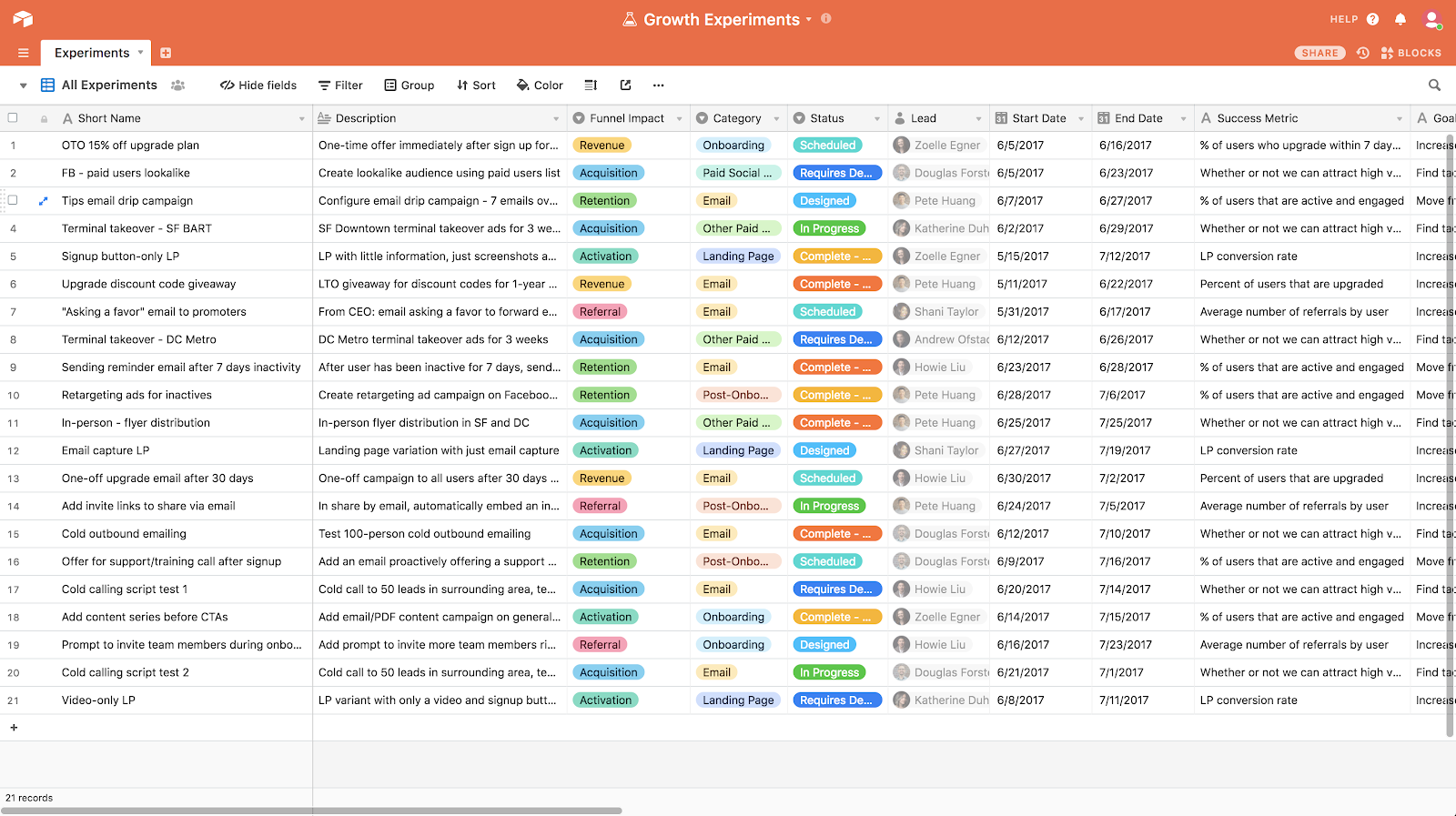
The Process
As with documentation, we take an iterative process for defining and prioritizing experiments. This process can be applied to both growth marketing and growth product experiments.
Hypothesis
It’s easy to justify running an experiment because another company is doing it in their app, but if our experiments aren’t based on our own hypothesis about our users and their customer journey, we’ll just be trying random things without a clear reason.
So we start by forming a hypothesis which states what we believe to be true about our customers. This hypothesis forms the basis of the experiment ideas we brainstorm and the overall design of our experiment.
The hypothesis also helps with having a set goal for the experiment. Why is it worth spending time running an experiment to prove this hypothesis is correct?
Brainstorming
We make it clear to everyone involved that there are no stupid ideas during a brainstorming session which results in everyone sharing ideas and withholding criticism (that comes later).
The hypothesis provides a guardrail for brainstorming sessions so it isn’t too open-ended. We also identify the metrics we want to improve so ideas are focused on moving that metric. To break it down further, if we understand all the inputs affecting the metric, we can brainstorm around those inputs as well.
For example, imagine you’re a product manager at Spotify and you want to increase the number of people buying tickets for local concerts. You might list the following pathways which lead to the desired action – booking a ticket:
- Open an email about a nearby concert, click the email to view the concert, purchase a ticket
- Listen to a song on Spotify, view artist page, view the artists’ Concerts tab, click to view upcoming concert, purchase a ticket
- Curious about local concerts, open Spotify, click on Concerts tab, click to view upcoming concert, purchase a ticket
Looking at only those three pathways, the brainstorming session could be based on getting more people to:
- Open and/or click the email about nearby concerts
- View the Concerts tab
- Click on an upcoming concert to get more details
(In this scenario, the website to purchase a ticket is a third party like Ticketmaster which Spotify can’t control.)
After the brainstorming session, we’ll briefly discuss each idea and come up with a short list of experiments which seem plausible. Each idea has a directly responsible person (DRI) and she is responsible for doing the research on the experiment.
Research
In the research phase, we dig into data, have conversations, and try to understand whether an idea has merit.
Research forms the skeleton for all our experiment documents because it informs our hypothesis, our objective statement, the experiment design, the predicted outcome, and what success looks like.
At HubSpot, research for an experiment could include creating charts in Amplitude, creating reports in Google Analytics, reviewing previous experiments, and conducting user interviews. The research process will look different depending on your business and the tools you use.
From our research, we’ll be able to determine the potential impact of improving a metric. We’ll also be able to start thinking up new experiment designs and developing an idea of how much effort will be required to run the experiment.
The effort might include:
- Aligning with other teams
- How much of the work would affect other teams?
- How many teams do we need to inform?
- Getting buy-in from stakeholders
- How much of the product and how many customers would it affect?
- Who needs to agree it’s worth doing?
- Technical limitations
- How involved are the technical challenges?
- Do we need to dig into another teams’ repository?
This is a point where we might decide, “This experiment doesn’t make sense. The upside is low, and it requires too much effort.” Or “We should definitely run this experiment. It’s low effort and potentially high impact.”
Objective Statement
In this section, we simply state why we’re running the chosen experiment. In general, it’s to improve some metric, which will result in down-funnel improvements, namely an increase in revenue.
Experiment Design
Once we’re clear on the hypothesis, we design an experiment that will determine whether or not the hypothesis is true.
The experiment design includes the number of variations we want to show, the copy and designs we want to use, who we expose the experiment to, and how we will track and measure the experiment, how long we will run the experiment, and the sample size required to reach statistical significance.
Keep in mind, a failed experiment does not necessarily mean the hypothesis is false. There are multiple ways to test a hypothesis. One failed experiment could simply mean a particular hypothesis is false in a specific instance.
Predicted Outcome
As mentioned above, we generally run experiments to improve some metric. However it’s easy to explain how an experiment could improve a simple metric such as click rate. The impact should always be translated into business growth ($$$) which determines the actual impact of an experiment.
So if we want to improve the click rate at some point of the experience, does that translate to more $$$? If so, how much?
An example predicted outcome:
If our hypothesis is true, we expect a 10% improvement in the click rate on the “learn more” button. Assuming all other conversion points remain constant, we expect this to translate into 20 more purchases per week. With an average sales price of $50 MRR, that translates to $4,000 new MRR per month.
You might be looking at other metrics such as new user signups to your freemium product.
Another example predicted outcome:
If our hypothesis is true, we expect a 5% improvement on the signup page form submission. Assuming traffic to the page remains constant, we expect this to translate into 100 more signups per day.
If you have more data such as upgrade rate and average sales price, you can translate it into revenue.
What does success look like?
As we go through the process of research and designing an experiment, it’s easy to get lost in the weeds and lose scope of why we wanted to run the experiment in the first place.
We always have a section in the experiment document that defines success. For example, success might be defined by improving a metric by a certain percentage or getting the answer to a question.
Peer Review
Once we have the complete experiment write-up, we share it in our growth team Slack channel for feedback.
Questions asked might include:
- What data do we have to support this hypothesis? Why do we believe this to be true?
- How does achieving this objective help us take a step toward achieving the company’s goals?
- How will we track performance? Is there another, less resource-intensive way to test the hypothesis?
- Is this the best metric to measure success?
- Is this prediction realistic?
We also suggest potential follow up experiments that will continue to test the hypothesis.
The team is incredibly smart and always has feedback. However, we avoid bike-shedding, a concept adopted from Patreon. We focus on shipping the experiment to learn rather than endlessly discussing trivialities which might cause bottlenecks.
Prioritize and Run
From our research and discussion, we get an idea of the potential impact and effort required to run the experiment.
Then we run the experiment and… 🤞🏼
Analysis
Once the experiment is complete, the experiment DRI analyzes the numbers, and shares learnings with the team which is presented at our experiment readout.
It’s important to avoid misusing data analysis to find patterns of data and presenting it as statistically significant, a process known as p-hacking.
It’s important to avoid misusing data analysis to find patterns of data and presenting it as statistically significant, a process known as p-hacking.
To avoid p-hacking, it’s important to have a solid experiment design by defining how long we’ll run the experiment and the sample size we need to collect, as well as being 100% transparent in how we analyze the data.
A few methods of p-hacking include:
- Stop collecting data once the desired result is obtained (where p < 0.05)
- Exclude data points to get the data to show the desired result (where p < 0.05)
- Transform the data to get the desired result where (p < 0.05)
There could be an entire essay written on p-hacking (in fact, there are), so here’s some more reading about p-value hacking:
- Experiments at Airbnb – Airbnb Engineering & Data Science – Medium
- P-Hacking, or Cheating on a P-Value – Freakonometrics
- False-Positives, p-Hacking, Statistical Power, and Evidential Value – Leif D. Nelson [pdf]
Post-Mortem Experiment Readout
We meet as a team each week to discuss experiment results and next steps.
Each experiment DRI talks through her experiment, recapping the hypothesis, the learning objective, why we decided to run the experiment, the results, the learnings, and next steps.
The team digs into the data and learnings and we spend time discussing the potential implications of the results. New experiment ideas often come from these discussions and we add those to the growing backlog of ideas.
These discussions help you find and track patterns in how people use your product based on different product experiences and designs.
I’ll admin, most experiments my team and I ran were failures, but we learned a lot and it meant that the successful experiments felt that much sweeter.
How can startups apply these principles?
It’s clear that HubSpot has a very robust experimentation program. It might seem heavy and most startups don’t need that level of rigor. Instead, you should be focused on getting experiments live and learning.
Don’t let documentation and process be a barrier to learning and progress.
Your Turn
What experiments do you have coming up? What challenges are you facing in running experiments?
Want to learn more about how a high-performing growth team tackles high-impact experiments? Check out this case study on Patreon’s onboarding.
Common Questions
How do we get started on experiments?
Have an engineer who can think as a product manager and marketer start digging into the data and running a handful of experiments. Be intentional but don’t bike-shed. Just start running experiments and learn.
How do we come up with experiment ideas?
Start with your goal. Think about the inputs needed to achieve the goal. Build a model and understand what points are most critical to improving. Ask what you can do to improve the conversion point.
How do we know what will work?
You don’t. That’s why you run experiments.